Researchers obtain new valuable insights on nanoscale friction via AI-based predictive model
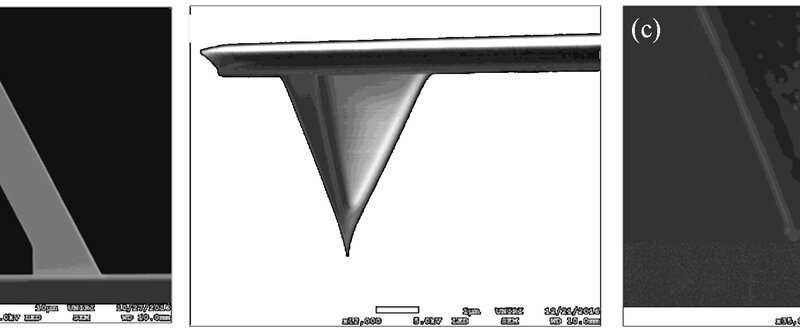
Friction has been an ever-lasting research problem, ever since the fundamental experimental observations of Leonardo Da Vinci, through the empirical laws postulated by Amontons and Couloumb and until today, when we still do not have appropriate understanding of this complex stochastic phenomenon. In fact, friction is omnipresent and has a substantial influence on everyday phenomena such as energy consumption—with some estimates suggesting that it results in costs of up to more than 1% of the global world's GDP, as well as on the wear of machine components, its influence on the precision of machines, etc.
In order to understand the behavior of two surfaces in relative motion, frictional phenomena have to be studied at multiple scales, down to the micro- and nanolevels and the level of molecular interactions. Even though a surface may look smooth, if it is magnified, it will clearly show asperities whose behavior under sliding contact presents a fundamental mechanism for the genesis of the macroscale friction force. What is more, friction is extremely difficult to describe using a model, especially in a form of a relatively simple and user-friendly mathematical expression.
How friction behaves with multiple variable parameters
The researchers from the University of Rijeka, Croatia, post-doctoral investigator Marko Perčić and
Professor Saša Zelenika, in collaboration with professor Igor Mezić from the University of Santa Barbara, California, U.S., recently conducted experimental measurements of nanoscale friction on various thin film materials via the lateral force microscopy (LFM) mode of a scanning probe microscope (SPM). SPM is a versatile instrument that can detect and measure miniscule forces (in the range of pN) resulting from the interactions between a nanometer-sized tip of the instrument and the studied sample.
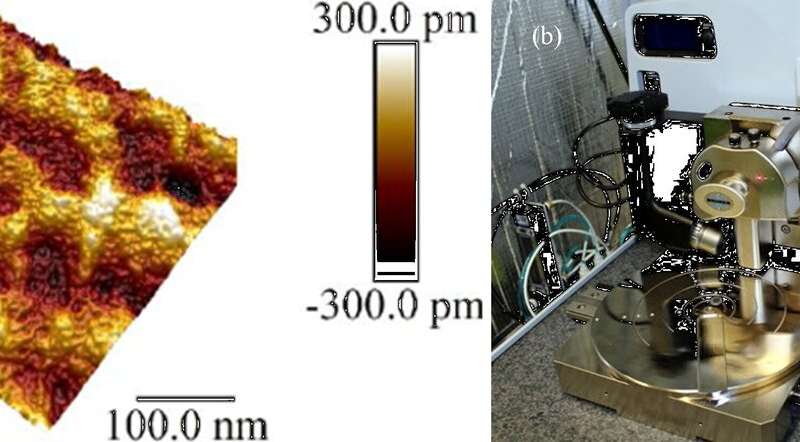
Perčić explains: "In a paper published last year in the Springer-Nature Friction journal, we describe a systematic experimental characterisation focused on technological thin films, based on elaborated Design-of-Experiments (DoE) methodologies, as well as on meticulous probe calibration and correction procedures, which allowed for the first time the determination of the values of nanoscale friction under the concurrent influence of several process parameters, comprising normal forces, sliding velocities, and temperature."
Mezić adds, "This study allowed providing an indication of the intricate correlations induced by these interactions and mutual effects. With the goal of overcoming the limitations of currently available models in ascertaining the effects of the physicochemical processes and phenomena involved in nanoscale contacts, our experimental measurements created the preconditions to undertake an effort to model friction in the nanometric domain."
![Figure 3. Predictive performances of the best developed AI (MG GP) model on the test dataset for (a) Al and (b) MoS2 with respective uncertainty levels in three shades of grey [Ref: https://doi.org/10.1007/s40544-021-0493-5 ]. Credit: Authors Researchers obtain new valuable insights on nanoscale friction via AI-based predictive model](https://scx1.b-cdn.net/csz/news/800/2021/6-researcherso.jpg)
Description of nanoscale friction with a simple mathematical expression
Perčić says, "In a study just published in the online version of the Friction journal, we have hence shown that machine learning (ML) and artificial intelligence (AI) methods can provide effective predictive tools for nanoscale friction, with rather good accuracy levels, but the intrinsic nature of black-box ML algorithms prevents their use in most practical applications. On the other hand, however, despite the marked complexity of the analyzed phenomena and the inherent dispersion of the measurements, the AI-based symbolic regression models that we successfully developed allowed attaining excellent predictive performance, with the respective prediction accuracy—depending on the sample type, between 72% and 91%".
Zelenika says, "This has allowed us also to attain an extremely simple functional description of the multidimensional dependence of nanoscale friction on the studied variable process parameters. A single and extremely simple mathematical expression, resulting in very high predictive performances, was thus finally obtained via an elaborated development based on multi-gene genetic programming (MG GP), enabling us to confirm the low impact of sliding velocity, a high positive impact of the total normal load, and a high nonlinear impact of temperature on nanometric friction."
![Figure 4. Surface plots of AI (MG GP) model results for constant variables in columns (left to right): normal load FL, sliding velocity v, and temperature θ, for the Al (top row) and MoS2 (bottom row) [Ref: https://doi.org/10.1007/s40544-021-0493-5]. Credit: Authors Researchers obtain new valuable insights on nanoscale friction via AI-based predictive model](https://scx1.b-cdn.net/csz/news/800/2021/7-researcherso.jpg)
Broad application spectrum and future possibilities
The resulting correlation functions, linking the considered process variables to the value of nanometric friction, provide a thorough insight into the phenomena of complex interactions, as well as a valuable, novel and unprecedented contribution in the field of nanotribology. Additionally, the assessment of an abundance of experimental results via testing based on state-of-the-art numerical methods, the resulting systematic evaluation of the predictive performances of these numerical methods, and, finally, the original proposed model with marked high-predictive performance and simple implementation, apt to be used for practical applications, are all important contributions of the performed work.
Perčić says, "All this constitutes the preconditions and provides means for an in-depth understanding and for practical improvements in the field of nanotribology, and a novel insight into this fundamental force of nature."
More information: Perčić, M., Zelenika, S., Mezić, I., Peter, R. and Krstulović, N. An experimental methodology for the concurrent characterization of multiple parameters influencing nanoscale friction. Friction 8(3), 577–93 (2020). doi.org/10.1007/s40544-019-0289-z
Perčić, M., Zelenika, S. and Mezić, I. Artificial intelligence-based predictive model of nanoscale friction using experimental data. Friction (2021). doi.org/10.1007/s40544-021-0493-5
Raw data used for training and testing the models is available at: github.com/mpercic-riteh/nanotribo
Marko PERČIĆ. He earned his D.Sc. degree in 2020 at the Faculty of Engineering of the University of Rijeka, Croatia. He is currently a postdoc at the Faculty of Engineering, Head of the Laboratory for Artificial Intelligence in Mechatronics at the Center for Artificial Intelligence and Cybersecurity, and a staff member of the Centre for Micro- and Nanosciences and Technologies of the University of Rijeka. His research interests are in experimental nanotribology, atomic force microscopy, thin films, mathematical modeling, and data mining.