Neural network taught to predict the quality of welding
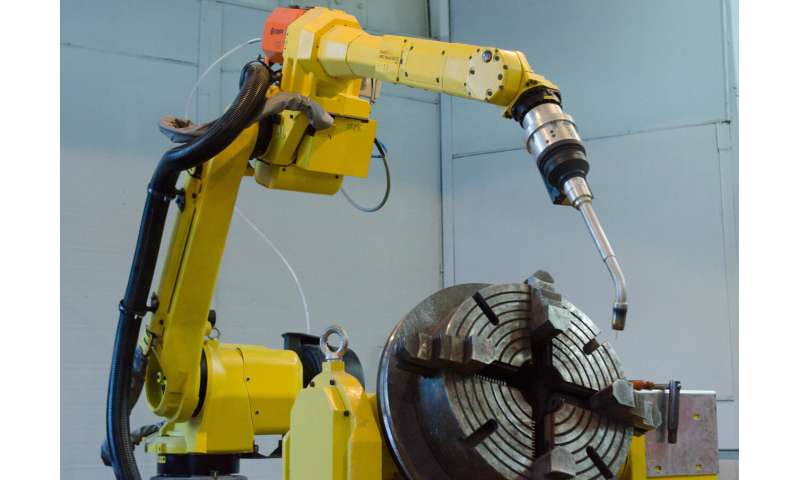
Artificial intelligence can evaluate the strength of a welding joint even before it is finally ready.
Scientists at South Ural State University have developed a neural network that can predict the quality of welds from fluctuations in current strength and other parameters of the welding process. An article on the characteristics of a regression model and an artificial neural network when monitoring welding quality is published in one of the highly ranked journals of the first quartile JMR&T.
As one of the authors, associate professor of South Ural State University (Chelyabinsk) Mikhail Ivanov noted, it is now very significant for the industry to be able to automatically evaluate the quality of welds. This is required by the fact that simple control does not allow you to perform this quite accurately.
Therefore, material scientists all over the world are trying figuring out how they can quickly evaluate the quality of welding both after it is completed and before it. This is important both to prevent possible problems during the operation of cars, appliances, and buildings and to save resources expended during welding.
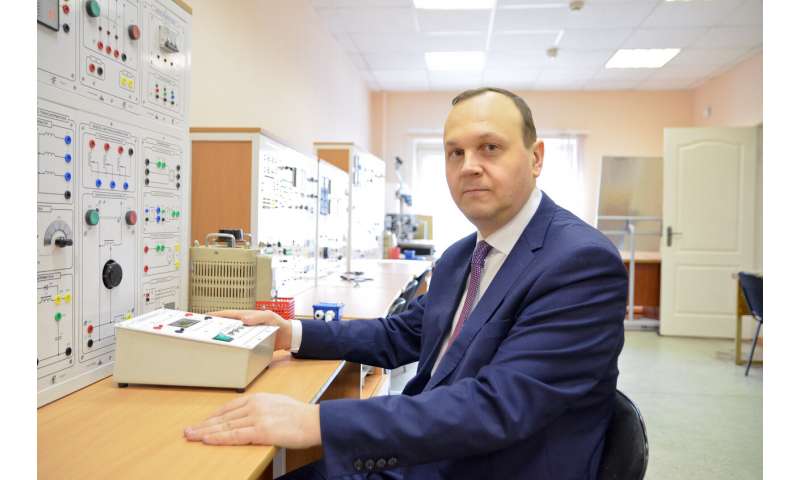
Neural Network Tips
Ivanov and his colleagues from China found out that this problem can be solved by operating a neural network. Resistance spot welding is a frequently used welding technique that has the advantages of low cost, a high degree of automation and a small workload. It is frequently used for joining steel sheets for car bodies and other large metal products, the electrodes of the welding machine and the workpiece interact with each other.
In such welding, workers or a robot apply electrodes to specific points on the surface of the parts being welded, pass current and compress them. At this point, the metal warms up much, melts, and a droplet-shaped welding joint fastening appears between its sheets.
As materials scientists explain, its quality depends on many parameters, including current strength, processing time, the pressure exerted on the sheets and how long they cool before the next welding session. All these factors influence each other; therefore, it is quite challenging to employ them to predict the quality of welds.
Chinese and Russian material scientists examined how the properties of the joint between two sheets of titanium changed. Researchers adjusted one or more of these parameters. Using this data, they trained the artificial intelligence system and also calculated the strength of the welding joints using methods of mathematical statistics. As a result, scientists constructed a neural network that can pretty accurately assess the quality of a welding joint even before it is ready.
As the researchers hope, their findings can be used in industry: employing these technologies, car manufacturers, as well as welders, will be able to work with metal faster and more efficiently, without sacrificing the strength of the structures made.
More information:
doi.org/10.1016/j.jmrt.2019.11.050
Provided by South Ural State University