Big data technology enables rapid online construction of battery open circuit voltage
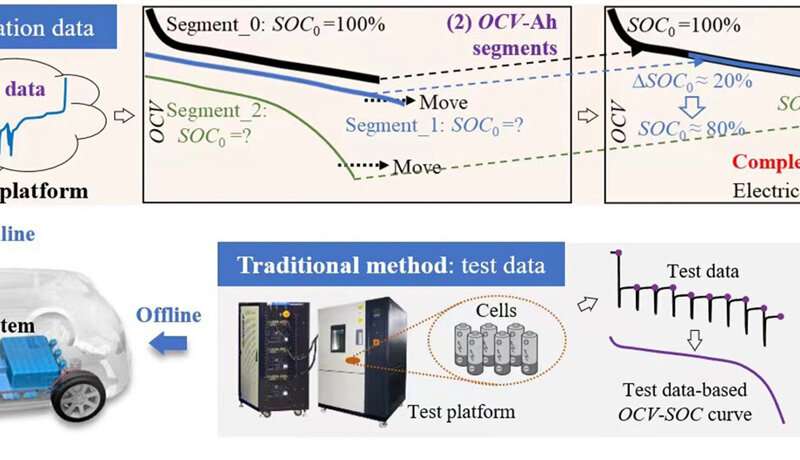
A paper outlining battery open-circuit voltage characterization was published in the journal Green Energy and Intelligent Transportation.
The battery management system (BMS) is crucial to the safety and reliability of electric vehicles (EVs) and battery energy storage systems. State estimations including SOC and SOH of lithium-ion battery (LiB) are foundational to BMSs. How to obtain the relationship between battery OCV and SOC is the key step in developing models for state estimation.
Over the last decade, researchers have built the OCV-SOC relationship is based on lab battery tests, which include the low-current OCV test, the incremental OCV test, and the dynamic condition test. The OCV-SOC relationship is usually affected by the battery temperature and aging degree.
To consider such influences, a series of OCV tests and aging tests need to be carried out to obtain a holistic OCV-SOC relationship, which is extremely time-consuming and costly. Furthermore, cell inconsistency is another key issue affecting the OCV-SOC relationship.
Generally, the real SOC can be calculated by the accumulation of electric quantity based on the measured battery capacity where the battery is fully charged and discharged. However, the batteries data measured on board are seldom fully charged and hardly fully discharged, the accurate SOC cannot be acquired using the ampere-hour (Ah) integration method.
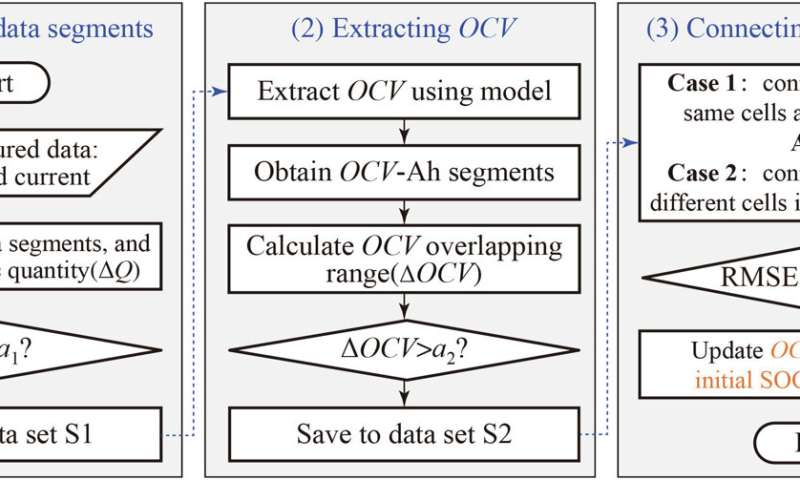
To obtain accurate real SOC, the researchers partitioned the operation data into data segments to reduce the influence of the accumulated error of electric quantity caused by uncertainties in data collection.
The researchers developed a data-driven method to construct battery OCV-SOC curves using onboard data. The procedure to construct the OCV-SOC curve can be divided into 3 steps: obtaining data segment, extracting OCV, and connecting OCV segments.
Firstly, the collected operation data includes the measured voltage, current, and temperature of batteries from the BMS or acquisition circuit; Secondly, by applying the least-squares algorithm to carry out the parameter identification for the data segments, the OCV sequence can be extracted from the raw voltage and current sequences; Thirdly, lots of OCV-Ah segments can be obtained by coupling the OCV sequence and the Ah sequence.
Because some factors, such as aging levels, operating temperature, driving conditions, and cell inconsistency can affect the OCV-SOC curve, they test the effectiveness of the data-driven method for batteries with different chemistries at different temperatures and aging levels. The results demonstrated far superior performance compared to the conventional way, achieving the maximum deviations are less than 3.0% for SOC and 2.9% for SOH respectively.
It has been found that the (root mean square error) RMSE, the length of the data segments, and the OCV overlapping range can clearly affect the error of the initial SOC. The initial SOC and SOH segment used to construct the reference OCV-SOC curve should be accurate, and accurate SOC data segments at different temperatures and aging states are also important to improve accuracy over the whole lifespan.
In future efforts, the researchers want to realize high-precision state estimation for batteries with different positive and negative electrode materials and further realize intelligent battery management.
More information:
Cheng Chen et al, A novel data-driven method for mining battery open-circuit voltage characterization, Green Energy and Intelligent Transportation (2022). DOI: 10.1016/j.geits.2022.100001
Provided by Beijing Institute of Technology Press Co., Ltd