Next-gen air safety systems incorporating risk models and data analysis
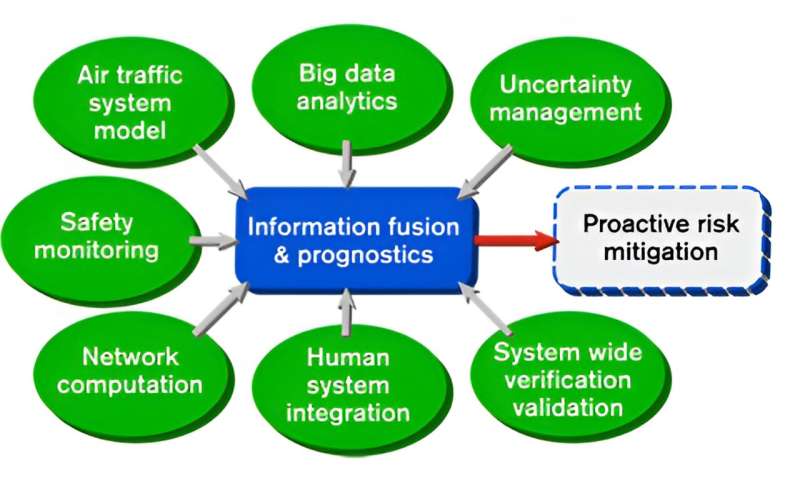
As the nation's skies become more crowded with commercial air traffic and U.S. air traffic control systems struggle with staffing and aging infrastructure, the risk of narrowly avoided collisions in the air and on the tarmac has increased significantly in the last five years.
The nation's top accident investigator said that a surge in close calls between planes at U.S. airports this year is a "clear warning sign" that the aviation system is under stress. "Our safety system is showing clear signs of strain that we cannot ignore," Jennifer Homendy, chair of the National Transportation Safety Board, told a Senate panel Nov. 9.
Most of the close calls are the result of human error, according to the U.S. Federal Aviation Administration. Controllers and pilots base their decisions largely on experience and judgment, and they generally do not rely on an autopilot system during takeoffs and landings, according to a veteran long-haul pilot.
Following the recent conclusion of a $10 million, five-year NASA grant, research conducted by experts from the Vanderbilt School of Engineering can be used to integrate risk models and relevant data sources into future air traffic management systems. The project was part of NASA's Aeronautics University Leadership Initiative that gives top academic centers and their industry collaborators a larger role in shaping best practices and translating them into commercial use. Industry partners Optimal Synthesis, Inc. and Southwest Research Institute (SwRI) joined Arizona State University and NASA to round out the Vanderbilt team.
Revolutionary technologies
When NASA originally launched the Transformative Aeronautics Concepts Program, the charge was to explore "revolutionary technologies, operational concepts, design tools, models, or other advancements we can't even begin to characterize today," according to program director Doug Rohn.
Yongming Liu, Ph.D. '06, ASU engineering professor, was the multi-team project lead investigator. The Vanderbilt team included Erin DeCarlo, Ph.D. '17, senior research engineer at SwRI. Sankaran Mahadevan, John R. Murray Sr. Professor of Engineering and civil and environmental engineering professor, and doctoral adviser to Liu and DeCarlo, led the Vanderbilt team.
The Vanderbilt task focused on diagnosis and prognosis of safety events during cruise, landing and takeoff. The team drew on its expertise in areas of validation and safety assurance as well as machine learning, uncertainty management, risk assessment and prognostics.
"Our work will have an impact on future air traffic management systems," said Mahadevan, an internationally recognized expert in reliability and risk engineering and computational modeling and co-director of Vanderbilt's Laboratory for Systems Integrity and Reliability. "It has been a consequential project because there has been an explosion of modern sensing, computing, machine learning and data science techniques, and this project harnesses these techniques to improve air travel safety."
The team stands at the forefront of global efforts in systematically advancing AI/ML techniques for air traffic anomaly detection and risk mitigation. The successful integration of historical knowledge, modeling and big data analytics in decision support for air traffic management has proven to be a highly effective approach, according to Liu.
"The multidisciplinary research training and system-level thinking I received at Vanderbilt University have profoundly influenced my approach to addressing complex problems. And reconnecting with former college friends and my adviser to work on something impactful for society has been truly gratifying," he said.
Key outcomes
In addition to new aviation courses and conference presentations, one of the key outcomes of the far-reaching research project was the development of an open-source simulation testbed called the Generalized National Airspace Traffic Simulation (GNATS). This platform, which is freely available on GitHub, offers gate-to-gate simulation involving flight vehicles in a national airspace system.
"NASA and the FAA have very active programs on aviation safety. We made solid contributions," said P.K. Menon, founder and CEO of Los Altos, California-based Optimal Synthesis Inc., the industry partner that built the GNATS platform used by the Vanderbilt researchers. "NASA has said this work is a 'model project' on which they will build."
For example, the team embraced the technical challenge of collaboratively developing, showcasing and distributing novel machine learning methods to the air traffic management (ATM) community. These contributions are housed side-by-side in the open-source toolset PARA-ATM and the PARA-ATM research collection managed by researchers and former students of Mahadevan's at SwRI.
"We were able to create a living legacy to the important work done by the team and, as a former Ph.D. student of Dr. Maha's, it was rewarding to help the students gain confidence in toolset development alongside their academic research," DeCarlo said.
The American Institute of Aeronautics and Astronautics (AIAA) has now offered a short course—AI for Air Traffic Safety Enhancement—that grew from the team's research. It includes an introduction to complex system safety modeling of multiple failures that include mechanical and electrical systems, miscommunications, human-automation errors, weather-related errors, and more.
Safety concerns grow
Air travel continues to grow rapidly. Estimates project that passenger volume will double by 2040, from 4 billion annually to 8 billion. "That means as air travel demands grow, adding more flights a day within the same airspace will certainly elevate safety concerns," Mahadevan said.
Aeronautic systems themselves have also become more complex and automated in recent years. The next generation national air transportation system is expected to have access to a multitude of new aviation data sources ranging from pilot-controller voice and data communications, higher-frequency airborne and surface surveillance data, high-frequency weather forecasts, and aircraft health data.
Mahadevan and Menon underscore the importance of prognostics modeling in aviation. The team used physics-based modeling and data-driven techniques to enable risk prediction and mitigation of adverse events. They developed tools that would give controllers and pilots a heads-up. "We want to analyze the data, develop models, and give them the predictions to help support their decisions," Mahadevan said.
"For example, I call a car's check engine light the idiot light," Menon said. "When that light came on, your engine likely is already wrecked. Wouldn't it be better to have a light indicate that within two weeks from now you had better see your mechanic? Prognostic information is the most useful information you can get."
Close call risks
To undertake the work, the Vanderbilt team consisting of two postdocs, three Ph.D. students and two undergrads combed through 20 years of National Transportation Safety Board (NTSB) accident investigation reports. The reports identify the flight phases—takeoff, landing and cruise—when accidents happen and analyze the causes of events.
What followed at Vanderbilt was careful construction of event sequences that include human errors, equipment failures, and weather effects as they unfold under real-world circumstances. In addition, data on 186 parameters available from the flight recorders, was used to construct machine learning models to predict the risks of different adverse events during landing.
"For controllers, this would identify the probability that, sequentially, if something went wrong, then this, this and this will go wrong, too," Mahadevan explained.
In addition to NTSB data, the team used safety reports from the Aviation Safety Reporting System (ASRS). ASRS is the FAA's voluntary reporting system that allows pilots, air traffic controllers, cabin crew, dispatchers, maintenance technicians, and ground operations to report confidentially any near misses or close calls in the interest of improving aviation safety. The models developed at Vanderbilt also used data from Houston (in-air separation violations), Detroit (landing safety), New York City and Atlanta airports (ground safety).
Out of that work, Mahadevan and his team developed new methods for analyzing and predicting risks related to the close call situations they examined. But before they get adopted in aviation, they will need verification, validation and certification, he added.
Mahadevan emphasized that commercial air flight remains the safest mode of travel. "Yet, with the growth in computational power, improvements in sensing, machine learning and statistical modeling, all contributing to a more robust understanding of how and why things go wrong, air travel can continue to be safe in the face of increasing demand."
Provided by Vanderbilt University