Integrated photonic convolutional acceleration core for wearable devices
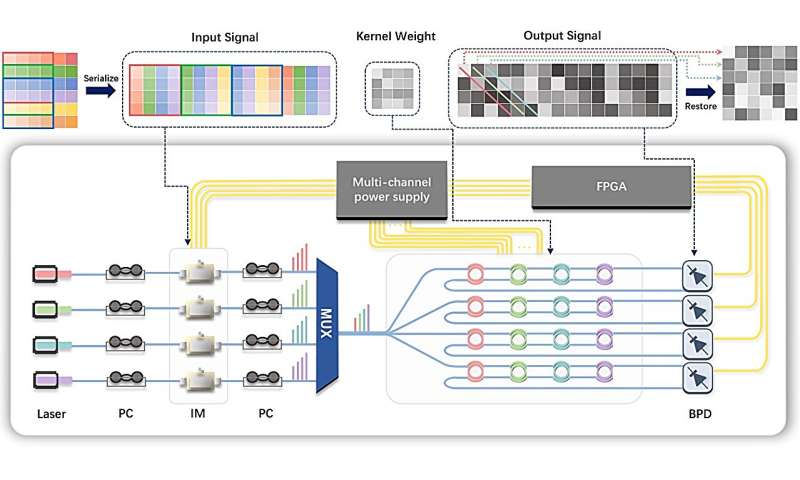
Wearable devices, characterized by their portability and powerful human-computer interaction, have long represented the future of technology and innovation. In the field of wearables, many recognition tasks rely on machine vision, such as vehicle detection, human pose recognition, and facial recognition.
These applications primarily rely on forward propagation of deep learning algorithms for classification and recognition tasks. However, with the increasing complexity of these applications, Moore's Law approaching its limits, and the increasing requirements of wearable devices for computational power, low power consumption, low heat generation, and high efficiency, traditional electronic computing is challenged, and it is imperative to investigate an alternative solution.
In recent years, optical neural networks (ONNs) research has emerged as a potential breakthrough solution to address the bottleneck of electronic computing. By mapping the mathematical model of a neural network onto a simulated optical device, optical neural networks can achieve computational power superior to electronic computing because optical transmission networks have the potential for ultra-low power consumption and minimal heat generation. This makes them well-suited to meet the energy consumption and heat dissipation requirements of wearable devices.
Some of the currently researched approaches are not very advantageous for meeting the requirements of future wearable devices due to some of their disadvantages in terms of integration and heat generation. In contrast, the array approach using micro-ring resonator (MRR) devices shows a greater potential for application in the field of wearable devices due to its compactness, ease of integration, and the ability to perform high-precision complex calculations through one-to-one assignments during parameter configurations, which makes it suitable for both small-size and large-scale applications.
This work presents a scalable optical convolution acceleration core (PCAC) based on reconfigurable MRR arrays with self-calibration capabilities. The system accomplishes the multiplication operation by multiplexing the multiwavelength optical signals with the weight matrix on the MRR, and the final computational results are obtained by weighted summation using balanced photodetectors (BPDs) under the self-calibrating MRR arrays the researchers have developed, and the convolutional results are obtained by reconstructing the output of the optical power difference.
Combined with field-programmable gate array (FPGA) control, the system is capable of performing high-precision calculations at the speed of light, achieving 7-bit accuracy while maintaining extremely low power consumption. It also achieves a peak throughput of 3.2 TOPS (Tera operations per second) in parallel processing. The findings are published in the journal Opto-Electronic Science.
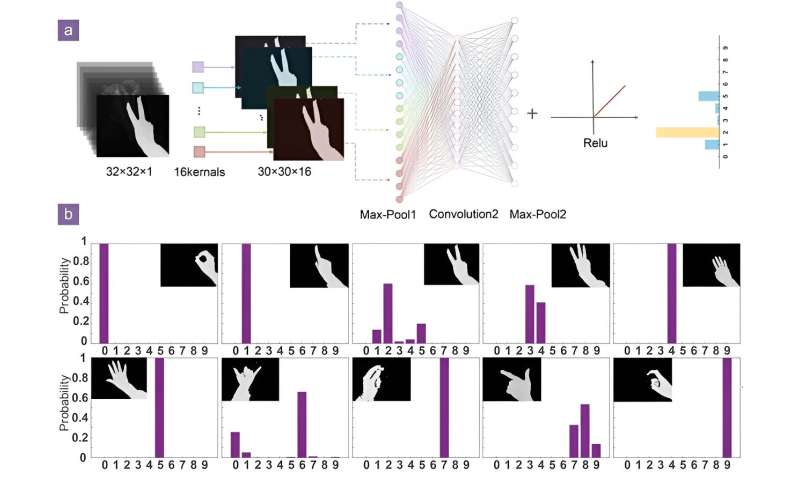
Based on this system, this work fabricated a proof-of-concept PCAC chip with 4×4 MRR arrays, and successfully used the chip for image edge extraction tests, as well as optoelectronic computation experiments in a typical wearable application such as AR and VR devices: first-person viewpoint gesture recognition based on depth information. By replacing electronic computation with parallel optoelectronic convolutional computation, the researchers realize efficient and highly accurate computation. Fig. 2(a) illustrates the main structure of the convolutional neural network (CNN) used in their application. The input image is reshaped into three rows of data and flows into the PCAC chip, where the first layer of the convolutional operation is performed entirely by the PCAC chip. Fig. 2(b) shows the probability bar graph of the recognition results of ten gestures computed by the PCAC chip.
In the 10 recognition samples of 0–9 digits, the recognition probability distributions of the remaining digits are all single peaks, except for the 2nd, 3rd and 8th digits, which have primary and secondary peaks. This indicates that the PCAC chip can accomplish the accurate recognition task. When the PCAC chip is used for photoelectric calculation, the recognition accuracy of all blind test images is the same as that of electronic calculation. It shows that the PCAC chip successfully realizes convolutional arithmetic and is fully capable of accomplishing wearable recognition applications with the advantages of low power consumption, high speed, and high accuracy.
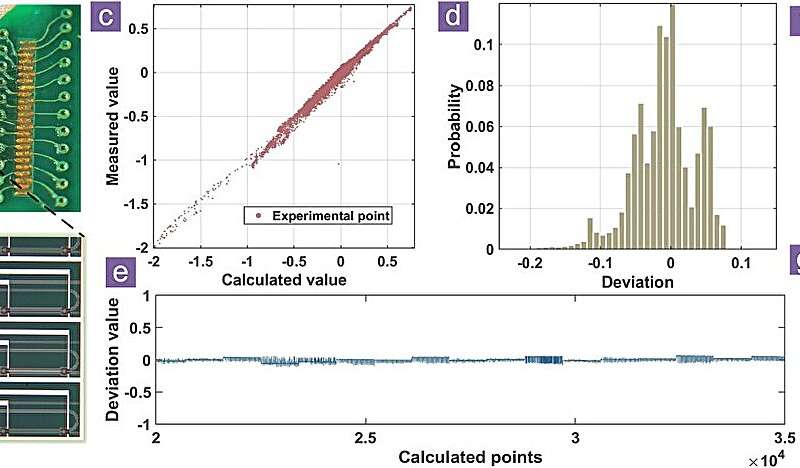
Figure 3 further investigates the performance of the PCAC chip in a computational task by comparing the experimental results obtained by performing convolutional computations using the PCAC with the theoretical results obtained by using a digital computer to recognize gestures 2. Except for some background color variations due to experimental noise, the results obtained from convolutional computation with the PCAC chip are almost identical to those obtained with the computer.
The analysis results show that compared with the theoretical results, the PCAC chip exhibits high accuracy and stability in the computational task, demonstrating its great potential as a gas pedal to perform recognition and classification tasks under highly low energy consumption conditions, and providing an effective optical solution for the further development of wearable devices.
More information:
Baiheng Zhao et al, Integrated photonic convolution acceleration core for wearable devices, Opto-Electronic Science (2023). DOI: 10.29026/oes.2023.230017
Provided by Compuscript Ltd