KD-crowd: A knowledge distillation framework for learning from crowds
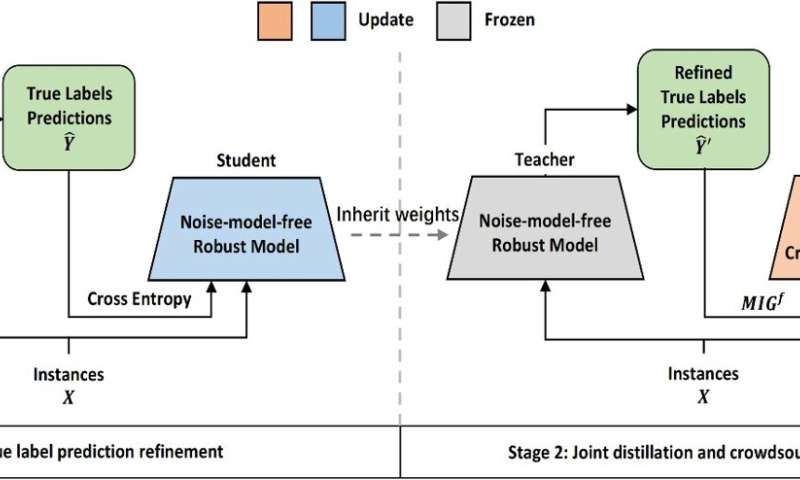
Crowdsourcing efficiently delegates tasks to crowd workers for labeling, though their varying expertise can lead to errors. A key task is estimating worker expertise to infer true labels. However, the noise transition matrix-based methods for modeling worker expertise often overfit annotation noise due to oversimplification or inaccurate estimations.
A research team led by Shao-Yuan Li published their research in Frontiers of Computer Science.
The team proposed a knowledge distillation-based framework KD-Crowd, which leverages noise-model-free learning techniques to refine crowdsourcing learning. The team also proposed one f-mutual information gain-based knowledge distillation loss to prevent the student from memorizing serious mistakes of the teacher model.
Compared with the existing research results, the proposed method demonstrated both on synthetic and real-world data, attains substantial improvements in performance.
Future work can focus on testing the effectiveness of this framework on regular single-source noisy label learning scenarios with complex instance-dependent noise and investigating more intrinsic patterns in crowdsourced datasets.
More information:
Shaoyuan Li et al, KD-Crowd: a knowledge distillation framework for learning from crowds, Frontiers of Computer Science (2024). DOI: 10.1007/s11704-023-3578-7. journal.hep.com.cn/fcs/EN/10.1 … 07/s11704-023-3578-7
Provided by Higher Education Press