Research aims to improve data quality in manufacturing, seeking 'golden data'
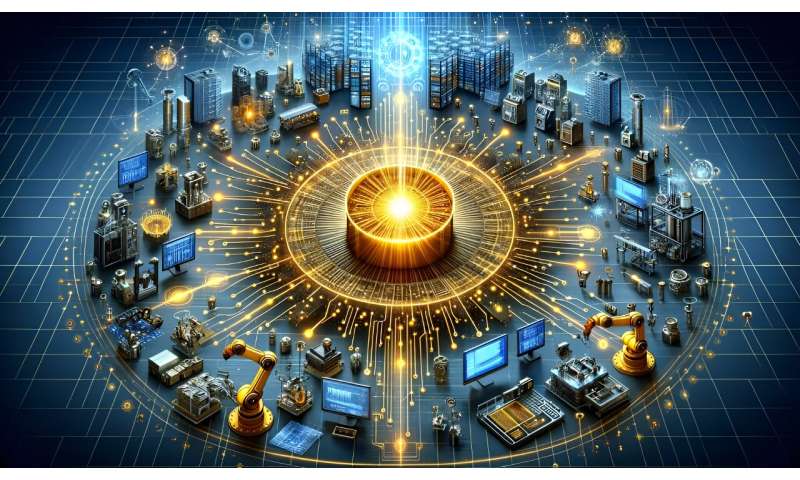
If artificial intelligence (AI) were a car, data would be the fuel. But what if there was no way to ensure that fuel wasn't full of waste? How would this fuel be filtered, and how would that information reach consumers?
Ran Jin, associate professor in the Grado Department of Industrial and Systems Engineering, is determined to fuel AI models in the Manufacturing Industrial Internet with high-quality data. His research aims to tackle three primary objectives:
- What is good-quality data? How is it defined?
- What is the root cause of bad data? Can it be prevented?
- Is there a "golden" data set that can be shared with other manufacturing processes and centers?
"The performance of machine learning and AI models pretty much depends on data quality. At this moment, there is no systematic way to define and evaluate data quality," Jin said. "We want to know: If we can define data quality and determine what causes poor data quality, can we improve it? If we can improve it, how can we share these data sets to improve AI development further? Then we plan to generate a golden data set which can be used across different platforms or systems."
Food for thought
Jin drew an analogy to cooking a turkey to explain the project's approach to improving data quality. Just as defining quantitative measures for a delicious turkey can lead to a better understanding of the potential reasons behind common cooking issues, this project aims to define and evaluate data quality quantitatively.
"Turkey is a pretty normal, ordinary dish, but it can be tricky to cook," Jin said. "If we evaluate how delicious a good turkey is, we must define a bunch of measures—whether it's juicy, crispy, salty, or has certain flavors like smokiness. That's essentially the first step."
When translating these factors to data, this might include how fresh, relevant, or complete the data is.
Once the markers for an ideal turkey are defined, the second step is to understand what didn't work. "Why did the turkey dry out? Why is the flavor off? It might be because we left it in the oven too long, or the seasonings weren't measured correctly: These are all potential root causes behind poor turkey turnout." Jin said.
Finally, Jin said the goal of his research is like sharing the perfect turkey recipe. Sharing this widely ensures a crispy, juicy, flavorful turkey every time—without unnecessary steps, ingredients, or waste. In a data quality setting, this translates to simplified, relevant, and useful data that can consistently inform machines and manufacturing systems to make good decisions.
"We want to determine how we can improve the turkey itself and optimize a recipe that works effectively and produces a delicious, perfectly cooked turkey that can be shared on the Internet with other cooks," said Jin. "With respect to data, we want to create a data set that can be effectively shared for AI development purposes, which has several merits such as representativeness, privacy protection, and effectiveness for AI model improvement."
Mapping outcomes
Despite the advances in manufacturing AI methodologies over the past decade, including significant strides in deep learning and neural networks, Jin points out that data generation and quality have become the major roadblocks in modeling and decision-making performance.
"More and more, people are realizing the bottleneck for overall modeling and decision-making performance is on the data generation and quality side," Jin said. "A typical phrase we use is 'put garbage in and get garbage out.'"
As AI becomes more advanced and widely used, ensuring access to high-quality data is crucial. Just as bad data quality can become a major barrier for improving decision-making in AI models, good data quality can pave the way for future advancements.
"In terms of broader impacts, data quality is the foundation for all types of research," Jin said. "While we're focusing specifically on electronics manufacturing in our research, this can be applied broadly to many different industries, like aerospace or biomanufacturing."
Breaking down the Manufacturing Industrial Internet
The Manufacturing Industrial Internet, as Jin describes, is key to collecting data from various manufacturing processes for adaptive computation for manufacturing improvements. This is different and more sophisticated than the internet, which connects our phones and laptops to the web.
The Manufacturing Industrial Internet connects everything in a manufacturing environment and is driven by AI instead of humans in decision-making. Additionally, it enables machines to communicate with other machines in a factory and a supply chain. The interconnected system can optimize quality, reduce cost and waste, and increase productivity and flexibility of product designs.
"The key functionality of the Manufacturing Industrial Internet is to collect data from different manufacturing processes and systems and use that information to provide real-time—or close to real-time—decision making and control," Jin said.
While the internet system we use regularly is not the same as the Manufacturing Industrial Internet, there are similarities between the two systems.
"Just like humans use an online social network to communicate with each other for better collaboration, the Manufacturing Industrial Internet acts like a social network of AI agents that communicate and collaborate with each other autonomously for different objectives," Jin said.
"Another example includes the Internet of Vehicles system connects vehicles on the road and transportation infrastructures or the smart grid—the system in which generators and distributors connect and talk with each other to make decisions and enable control collectively for better overall performance."
The future of AI success: Data quality
The Manufacturing Industrial Internet and AI have large quantities of data at their disposal. Jin hopes his research will better enable other data scientists to understand what is and is not useful.
"We have massive, passively connected data with very low cost from Manufacturing Industrial Internet. The question is: should we use all data or should we just use a smaller but more meaningful data subset?" Jin said. "The latter has several benefits, including lower computational workload and storage space, and it ensures better AI performance."
By enhancing data quality, the project not only aims to improve the efficiency and effectiveness of manufacturing processes but also sets the stage for broader applications across various industries, underscoring the profound impact of high-quality data on the future of manufacturing and beyond.
"I strongly believe this project will set the foundation to evaluate data. We cannot emphasize enough how important data valuation and quality is," Jin said. "Data is fuel for AI, and that is incredibly important for our future economy."
Provided by Virginia Tech