Machine-intelligence discovers new two-dimensional charge density wave materials
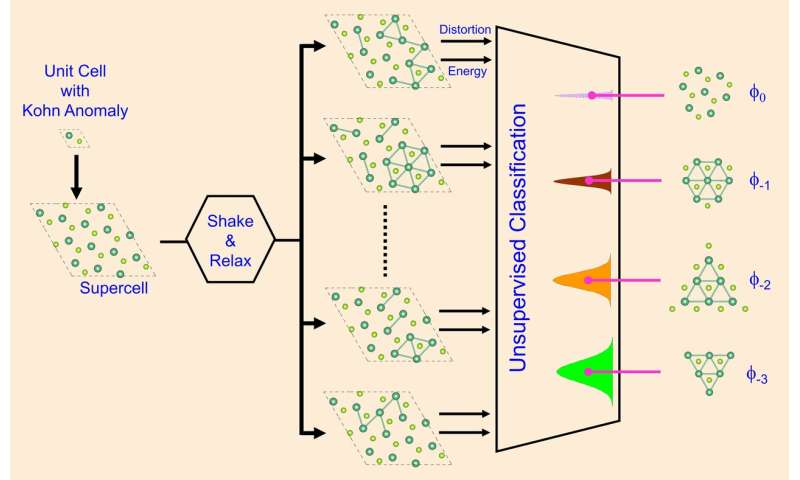
A crystal is a solid material whose constituents atoms are arranged in a periodic fashion. A charge density wave (CDW) is a quantum mechanical phenomenon observed in some crystals with metallic properties. It is usually characterized by a distortion in the periodic arrangement of atoms. This, in turn, results in a standing-wave-like periodic distortion in the otherwise uniform charge (electron) density, and hence the name charge density wave.
R.E. Peierls showed in 1930 that for a hypothetical one-dimensional chain of atoms with metallic nature, a sort of instability is induced due to a phenomenon called Fermi surface nesting. It leads to periodic distortions of metal atoms, which increases the energetic stability of the 1-D material with an opening of bandgap, i.e., the material turns from metal to insulator.
Subsequently, Walter Kohn mapped this to the vibrational spectrum of atoms (a.k.a. Phonon spectrum). He showed that below a critical temperature TCDW, the metallic material must show at least one sharp dip in its phonon spectrum, which is called the Kohn anomaly, with imaginary frequencies that signify dynamic instability. However, above TCDW, these imaginary frequencies disappear, indicating the stability of the metallic phase. The insulating phase, however, does not show any Kohn anomaly with imaginary frequencies even below TCDW. To summarize, an ideal CDW material shows the following properties:
Below TCDW:
- Periodic distortion in lattice and charge density.
- Energetically more stable than the "normal" or undistorted metallic phase.
- Insulating nature, i.e., bad conductor of electric current.
- No Kohn anomaly or imaginary frequencies in the phonon spectrum.
Above TCDW:
- The "normal" or undistorted phase is dominant.
- No periodic distortion, uniform lattice, and charge density.
- Metallic nature, i.e., good conductor of charge.
- Kohn anomaly could be there, but no imaginary frequencies in the phonon spectrum.
This electrical resistivity switching property of CDW materials is a very lucrative aspect for electrical engineers, as this is the main working principle of a transistor, the building block of processors of electronic gadgets. Although the CDW transition is defined by a change in temperature, this change can also be induced using other forms of energy such as a suitable voltage, current or light pulses. This led to the engineering of novel nanoelectronic devices using CDW materials, primarily TaS2, a quasi-two-dimensional CDW material that shows CDW transitions beyond even room temperature.
On the other hand, the downscaling of integrated transistors in processors has significantly slowed in recent times as engineers have reached a physical ceiling, indicating the eventual demise of Moore's law. Since the discovery of graphene, an atomically thin 2-D sheet of carbon, scientists and engineers all over the world have been trying to integrate these materials in nanoelectronic devices to allow further miniaturization of devices and also exploiting several novel and exotic properties of these materials to design devices based on different physics. Recently, several novel nanoelectronic devices such as oscillators and memristors have been designed using 2-D CDW materials.
However, the 2-D CDW materials deviate from the ideal 1-D behavior in a couple of ways. First, there is a co-existence and competition between several CDW phases, all of which are energetically densely situated. There have also been demonstrations of "hidden" CDW phases that are thermally inaccessible but can be invoked using electrical or optical excitations. Second, the bandgap opening does not occur in all CDW phases, i.e., most of the CDW phases show metallic behavior, albeit the electrical conductivity of the CDW phases is usually lower than that of the normal uniform phase.
Therefore, the design of 2-D-CDW based devices requires extensive knowledge of the CDW phases including the hidden phases, their energetics, and their electronic properties. The first principles-based methods are known for their predictive power and thus can be ideal for this purpose. However, these tools have so far been used as an analysis tool for the experimental data, rather than being employed as a predictive tool.
The rapid computational screening for exotic and application-specific properties in 2-D materials (e.g., non-trivial topological order, high-temperature ferromagnetism, superior catalytic activity, etc.) is becoming increasingly important. First-principles-based rapid prediction of CDW phases in 2-D materials has not yet been reported, probably because of its huge computational cost and subsequent requirement of manual inspection for phase-finding. Currently, there is a gaping scarcity of suitable 2-D CDW materials for nanoelectronic device applications. We bridge this gap by developing an automated high-throughput computational tool combining first-principles-based structure-searching technique and unsupervised machine learning, which identifies CDW phases from a unit cell with inherited Kohn anomaly and metallic nature.
We first selected suitable materials with Kohn anomaly and metallic nature from a database of 2-D materials easily exfoliable from their bulk parents. Then the unit cell of the material was multiplied to form supercells to capture periodicities of CDWs. After that, each atom in these supercells was perturbed in some random direction with some random magnitude. Then, these randomly distorted structures were relaxed to their nearest energy minima using density functional theory (DFT) calculations.
These energy minima for the distorted structures usually lead to either some CDW phase or the normal uniform phase. This whole process is termed as "shake and relax." extreme care is required while doing this, as the phases are energetically very close, and finding them requires accurate calculations, which in turn demands very high computational resources. We used hardware accelerators in this work extensively to satisfy this requirement. For each supercell (periodicity), we explored about 100 random distorted structures to sample the energy landscape satisfactorily. We performed this for more than 30 shortlisted materials, while three to four periodicities for each material were explored.
After the whole process, we obtained thousands of relaxed distorted structures and their energies. In studies focusing on a few materials, manual inspection led by experimental data is usually used to identify the CDW phases. In our case, this becomes impossible due to the sheer volume of the obtained data. Also, manual-inspection-based phase identification can be error-prone, and experimentally unexplored "hidden" phases could be missed. Instead, we used unsupervised machine learning to identify clusters in the energy-distortion space. Each cluster represents a phase, and the phase situated nearest to the cluster center is taken as the representative phase of that cluster. We used the "mean-shift" clustering algorithm for this task, which has found extensive use in the computer vision and image processing.
Although machine learning techniques are now widely used in material sciences, these studies mostly involve supervised regression to predict certain material properties. Here, we deployed unsupervised classification to replace the tedious and often error-prone process of manual phase finding, thanks to which we found a host of undiscovered phases for 2-D CDW materials such as 1T-TaS2 and 2H-NbSe2.
The proposed methodology not only rediscovers the known CDW phases, but also predicts a host of easily exfoliable "new" CDW materials (a total of 30 materials and 114 phases) along with associated electronic structures. Among many promising candidates, we paid special attention to ZrTiSe4 and conducted a comprehensive analysis to gain insight into the quantum-mechanical Fermi-surface-nesting, which causes a significant semiconducting gap opening in its CDW phase. We found a total of six 2-D-CDW materials with a significant bandgap opening in the most stable CDW phase, indicating that these materials would show an insulator-to-metal transition upon application of energy.
Our findings could provide useful guidelines for experimentalists and may foster the practical application of CDW materials in nanoelectronics.
This story is part of Science X Dialog, where researchers can report findings from their published research articles. Visit this page for information about ScienceX Dialog and how to participate.
More information:
Arnab Kabiraj et al. Machine-Intelligence-Driven High-Throughput Prediction of 2D Charge Density Wave Phases, The Journal of Physical Chemistry Letters (2020). DOI: 10.1021/acs.jpclett.0c01846
Bio:
Arnab Kabiraj is a PhD scholar at Indian Institute of Science, Bangalore, India. His present research activities focused on developing high-throughput computational framework for discovering 2D materials with specific properties.
Santanu Mahapatra is working as a professor at Indian Institute of Science, Bangalore, India. His research interests include two dimensional channel transistors, energy efficient electronic switches and energy-storage at nano-scale. [santanu.dese.iisc.ac.in]