A step towards personalized cancer vaccines
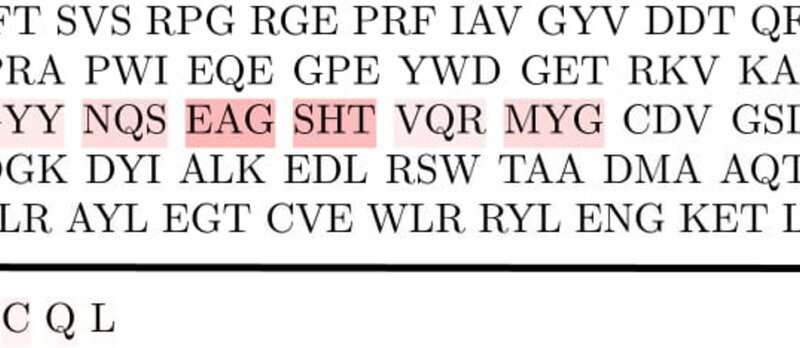
According to the World Health Organization (WHO), cancer is the second leading cause of death worldwide and was responsible for the death of an estimated 9.6 million people in 2018. Research is now focused on personalized cancer vaccines, an approach to help a patient's own immune system to learn to fight cancer, as a promising weapon in the fight against the disease. The immune system cannot easily distinguish between a healthy and cancerous cell. The way personalized cancer vaccines work is by externally synthesizing a peptide that, when administered to the patient, helps the immune system identify cancerous cells. This is done by forming a bond between the injected peptide and cancerous cells in the body. Since cancerous cells differ from person to person, such an approach requires analysis to choose the right peptides that can trigger an appropriate immune response.
Barriers
One of the significant steps in the synthesis of personalized cancer vaccines is computationally predicting whether a given peptide will bind with the patient's Major Histocompatibility Complex (MHC) allele. Peptides and MHC alleles are sequences of amino acids; peptides are shorter versions of proteins and MHC alleles are proteins essential for the adaptivity of the immune system.
A barrier to the smooth development of personalized cancer vaccines is the lack of understanding among the scientific community about how, exactly, the MHC-peptide binding takes place. Another is with the need for clinically testing different molecules before the vaccine is built, which is a resource-intensive task.
Approach
My colleagues and I at the International Institute of Information Technology Bangalore now present a new deep learning method called MHCAttnNet, which uses bidirectional-long short-term memory cells (Bi-LSTMs) to predict the MHC-peptide binding more accurately than existing methods. Our approach is unique in that it not only predicts the binding more accurately, but also highlights the subsequences of amino acids that are likely to be necessary to make a prediction. This research was presented at the 28th Conference on Intelligent Systems in Molecular Biology and is published in the Bioinformatics journal of the Oxford University Press.
We also use the attention mechanism, a technique from natural language processing, to highlight the essential subsequences from the amino acid sequences of peptides and MHC alleles that were used by the MHCAttnNet model to make the binding prediction. Suppose we see how many times a particular subsequence of the allele gets highlighted with a specific amino acid of the peptide. In that case, we can learn a lot about the relationship between the peptide and allele subsequences. This would provide insights on how the MHC-peptide binding actually takes place. Through MHCAttnNet, we show that the number of trigrams of amino acids of the MHC allele that could be of significance for predicting the binding, corresponding to an amino acid of a peptide, is plausibly around 3% of the total possible trigrams. This reduced list is enabled by what we call sequence reduction, and will help reduce the work and expense required for clinical trials of vaccines to a large extent.
Resources Used
Our work was supported by an AWS Machine Learning Research Award (https://aws.amazon.com/aws-ml-research-awards/) from Amazon. We used the AWS Deep Learning machine instances that come pre-installed with popular deep learning frameworks. It was a big help that we were able to quickly set up and use high-end machines on Amazon's AWS cloud for our sophisticated and custom deep-learning models, and easily experiment with new algorithms and approaches. It would have cost a fortune to own and operate such hardware outright, and this work is also an illustration of how artificial intelligence and machine learning research using cloud-based solutions can make a mark in different domains including medicine, in a much shorter time and at a fraction of the usual cost.
Are We There Yet?
We believe that this research will help other researchers in developing personalized cancer vaccines by improving the understanding of the MHC-peptide binding mechanism. The higher accuracy of this model will enhance the performance of the computational verification step of personalized vaccine synthesis. This, in turn, would improve the likelihood of a personalized cancer vaccine that works on a given patient. Sequence reduction will help to isolate a particular few amino acid sequences, which can further facilitate a better understanding of the underlying binding mechanism. Personalized cancer vaccines are still some years away from becoming a mainstream treatment for cancer, and this study offers several directions through sequence reduction that could make it a reality sooner than expected.
This story is part of Science X Dialog, where researchers can report findings from their published research articles. Visit this page for information about ScienceX Dialog and how to participate.
More information: [1] WHO Fact Sheet: Cancer (2018). www.who.int/news-room/fact-sheets/detail/cancer
[2] Gopalakrishnan Venkatesh et al. MHCAttnNet: predicting MHC-peptide bindings for MHC alleles classes I and II using an attention-based deep neural model, Bioinformatics (2020). DOI: 10.1093/bioinformatics/btaa479
Bio:
I am pursuing an integrated masters in Computer Science Engineering with a specialization in Data Science at the International Institute of Information Technology Bangalore, India. I started my final (fifth) year in August 2020. My research interests are using machine learning and artificial intelligence in the area of molecular biology. More details about me can be found on my personal website - aayushgrover.github.io/