Artificial intelligence discovers long-term influencers hiding in noisy systems
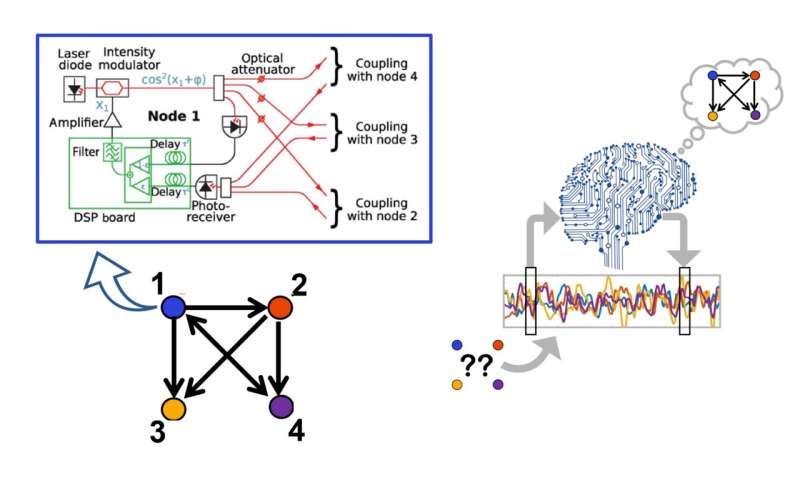
They say in chaos theory that a butterfly flapping its wings in Brazil could unwittingly set up a tornado in Texas. Well, maybe it does. But that tornado should at least need some time to form, given the 5,000-mile distance between the two regions. This time delay between cause and effects in climate patterns is well apparent in the less-dramatized example of El Niño events (as explained in this video).
These events occur roughly every two to seven years. But when they do, they build up over several months and their effects can take several months more to spread around the world. In a complex system like the global climate, the time delay between cause and effect makes it harder to pin down the key players influencing the system. The task becomes even more difficult when the system is noisy, like a whimsical and unpredictable weather pattern. But now, we have a way to uncover these long-term causal interactions—by only looking at how the system behaves over time.
Identifying time-lagged causal interactions is a key part in understanding and controlling the behavior of many complex systems found in nature and society. El Niño is just one of these systems, and there are lots of others. Even the humble act of turning the shower knob as we adjust for the perfect water temperature forces us to think about time delays. Ecosystems sustain themselves on feedback loops formed by myriad interactions between species, such as predation, coexistence and human interventions. Even a small-sized ecosystem with about 20 species can have thousands of such interactions, and together, they form a network (as shown in this video).
But the interactions depicted by the network links can be time-delayed—like overuse of pesticides can bring back insects in the long run, as shown in the video. Neurons in the brain gather electric signals from other neurons for some time before they decide to fire. Pandemics like COVID often spread through human-to-human contacts. But viruses spend a latent time in their hosts' bodies, making the spreading process time-delayed. Just as the contact tracing of COVID patients is important to stall the pandemic, identification of time-delayed interaction networks between different parts of any system is a key to the understanding of that system.
In order to trace such time-lagged interaction networks from the behavior of a system over time, we have used an artificial-intelligence-based technique. Our proposed method is two-step. First, we train a neural network to follow and mimic the behavior of the system (see figure). For example, a neural network trained to mimic a bunch of neurons will reproduce their firing patterns. Then, we propose to use that trained neural network model as a proxy computer model of the original system. That way, it becomes possible to zoom inside the neural network, play with its specifications, and ultimately check how different parts of it are connected. Since that already gave us a working model of the actual system, those connections can be directly mapped to causal interactions of the system itself, even if they are time-lagged. This reveals the long-term influences of one part of the system on another part. For example, it can reveal how the neurons in your brain are connected, how a snowstorm in my city affects the temperature of nearby cities, and the identity of that super-spreader who made every attendee sick. Once we get to know all these interactions, we can pinpoint the key "influencers" driving the behavior of any complex system, and use that information to better understand, predict, or control the system in general.
We tested whether this idea was working in our lab—we made a network out of four opto-electronic elements that were connected by delayed feedback. We sampled the voltages of those four elements (or four "network nodes") over time, put that data into our neural network, and tried to see if we could reconstruct the original network we had created in the lab just by looking inside the neural network. As it turned out, we could do that perfectly in most cases, with a caveat: The catch was that the voltages from those four nodes cannot be synchronized with one another.
Synchronization is a common phenomenon in nature (here is a video of synchronization of five metronomes coupled via a common moving platform), and is possible whenever multiple systems are coupled together. When the voltages of the four nodes were in sync, they could not be told apart from each other, and, quite expectedly, we could not gain any information of their underlying network. However, noise in our experiments, which hindered synchrony in general, helped us a great deal. Since most natural systems contain multiple sources of noise, in general, we hope that our technique will be useful for a great number of these systems
So we designed and tested a technique to understand how different time-lagged feedback loops and networks underlie the behavior of a multi-component system. Looking ahead, we plan to apply this technique to discover such influence networks in natural and artificial systems—ranging from brains, ecosystems, global climate patterns and stock markets. Our work thus opens a window to gain new insights about long-term influencers and influences in complex systems.
This story is part of Science X Dialog, where researchers can report findings from their published research articles. Visit this page for information about ScienceX Dialog and how to participate.
More information:
Amitava Banerjee et al, Machine Learning Link Inference of Noisy Delay-Coupled Networks with Optoelectronic Experimental Tests, Physical Review X (2021). DOI: 10.1103/PhysRevX.11.031014
Amitava Banerjee is a graduate student in the department of Physics, at the University of Maryland, College Park. His works include using machine learning to predict and analyze dynamical systems and networks.