Machine-learned phenomenological model for synthesis of inorganic crystalline materials
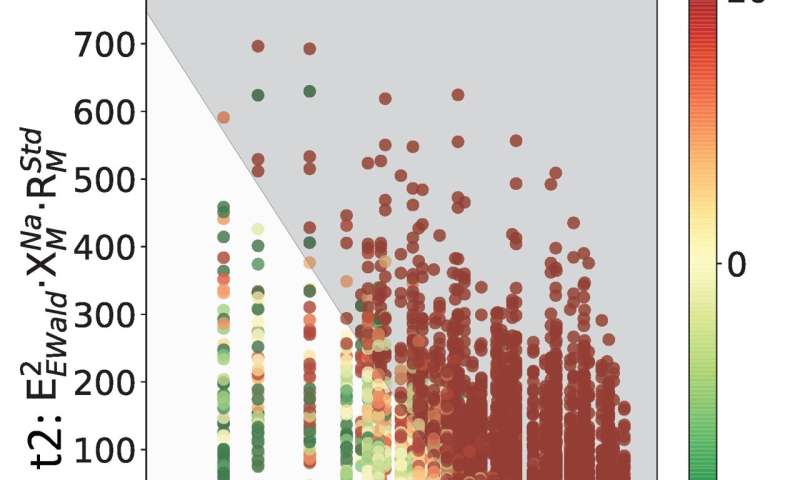
The establishment of materials science should acknowledge the contribution of phenomenological models. A few examples include: the famous Arrhenius relation that reveals the temperature-dependent kinetic process, and Fick's law that describes the mass diffusion along certain gradients. When considering the synthetic accessibility of new materials—that is, whether a designed material can be synthesized or not—we also benefit from models like the Goldschmidt tolerance factor, which describes the stability of cubic perovskites (chemical formula: ABO3). With an empirical rule t = (r_A+r_O)/√2 (r_B+r_O ) where r is the ionic radius, a stable, or synthesizable, perovskite should simply satisfy that t is close to 1. Recently, the increasing demand for renewable energy technologies has triggered the development of new energy materials, in which such tolerance factors would greatly speed up the discovery of new materials.
However, even with decades of development in materials science, we are still short of tolerance factors for most of the crystalline materials, mainly due to their complicated bond topology and compositional correlation. Such complexity inhibits the establishment of physical intuition on how materials can be stabilized or synthesized.
Fortunately, the process can be facilitated with symbolic machine learning techniques. We have applied sure independent screening and machine-learned ranking to identify tolerance factors for one group of complicated but useful oxide materials: NASICON solid-state conductors. NASICON materials have a rigid polyanion framework, within which Na ions are highly mobile; therefore, it can serve as solid-state electrolyte material for next-generation solid-state batteries.
In this work, we have performed high-throughput phase diagram calculations for 3881 potential NASCIONs and developed a tolerance factor for NASICONs. The simple relation, 0.203 × t1 + t2 ≤ 0.322 in which t1 and t2 is calculated from elemental electronegativity, ionic radius, ion charge states, Na composition and Madelung energy, can efficiently separate stable NASICONs from unstable ones.
With a combination of theoretical and experimental work, we have predicted 641 synthetically accessible NASICONs and had a 5/6 success rate of experimental synthesis. An interactive version of a comprehensive stability map for this kind of material is also released online at https://bin-ouyang.com/blog/NASICONStability.html. It provides direct guidance as to which elements are compatible and can therefore form a stable compound within this crystal framework.
Since the first report of NASICONs in the 1960s, during which most new NASICON materials were discovered in relatively narrow compositional space, we have expanded our knowledge of NASICON stability to a much wider chemical space by high-throughput computation and a machine-learning phenomenological model. With our work on NASICON, we have demonstrated that machine learning can not only serve as a "black box" for accurate predictions, but also can be used to discover new scientific theories.
This story is part of Science X Dialog, where researchers can report findings from their published research articles. Visit this page for information about ScienceX Dialog and how to participate.
More information:
Bin Ouyang, Jingyang Wang et al. Synthetic accessibility and stability rules of NASICONs, Nature Communications (2021). DOI: 10.1038/s41467-021-26006-3
Dr. Bin Ouyang is a theorist working on the edge among materials science, data science and machine learning. He obtained PhD in Materials Engineering at McGill University in 2017. Currently, he is a postdoc scholar at University of California Berkeley working with Professor Ceder. He has published more than 45 journal papers on data driven materials discovery. More details about him can be found on bin-ouyang.com/.
Dr. Jingyang Wang is a postdoc researcher at University of California, Berkeley supervised by Professor Gerbrand Ceder. His research mainly focuses on the development of electrode and solid-state electrolyte materials for next-generation beyond-Li batteries. He obtained his PhD in Materials Science and Engineering at University of California, Berkeley in 2021.